A multi-modal modified feedback self-paced BCI to control the gait of an avatar 논문 리뷰
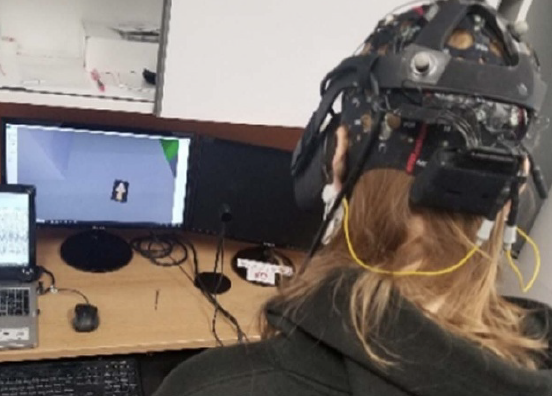
본 논문은 virtual reality avatar의 single steps와 forward walking을 control할 수 있는 multi-modal BCI를 제안한다.
Materials and methods
A. Data acquisition and recording
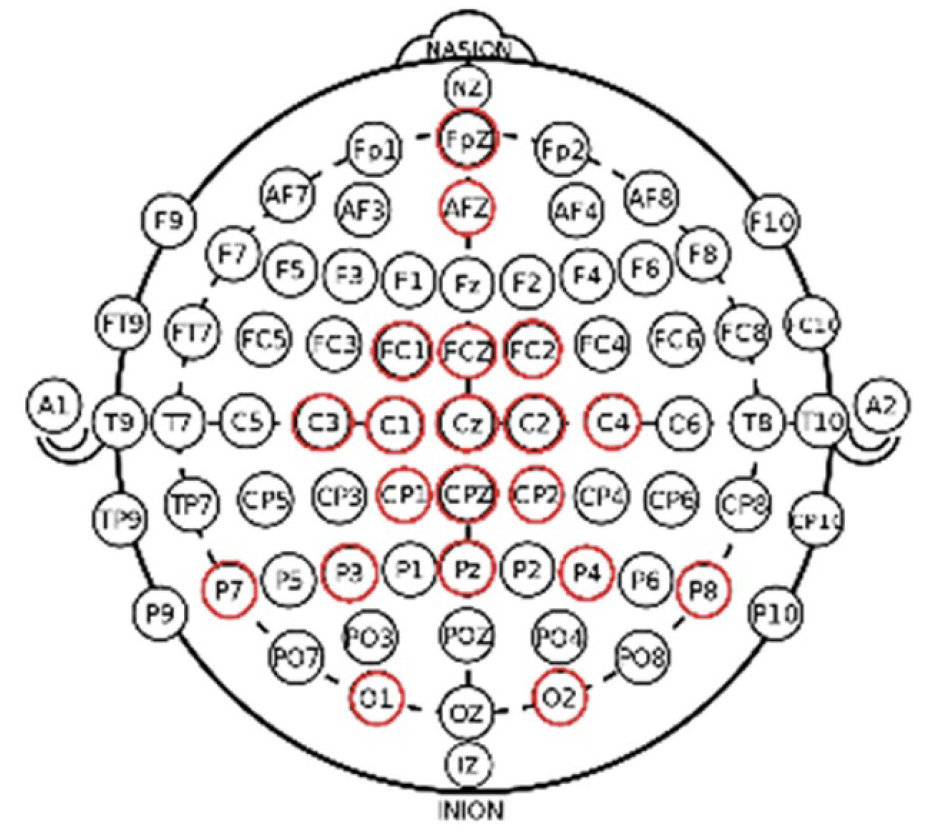
EEG data는 위와 같이 추출되며 AFz 전극이 ground로 사용된다.
이 data는 EEGStudio를 통해 stream되었다.
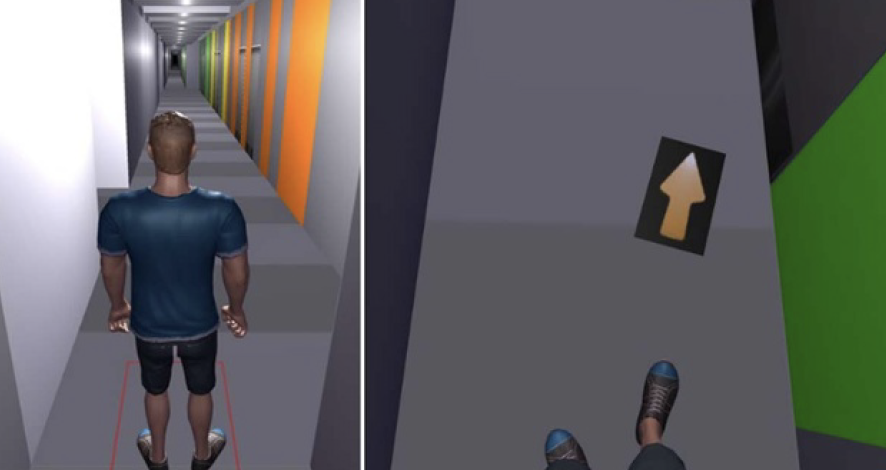
Virtual Environment(VE)는 Unity3D로 구현되었고 Avatar의 경우 MakeHuman을 통해 만들어졌다. 이 avatar가 걷는 모션은 Mixano를 통해 얻어졌다.
VE는 위와 같으며 avatat의 움직임을 인지시키기위해 벽에 수평선이 있다. (주황, 초록색)
B. Experimental protocol
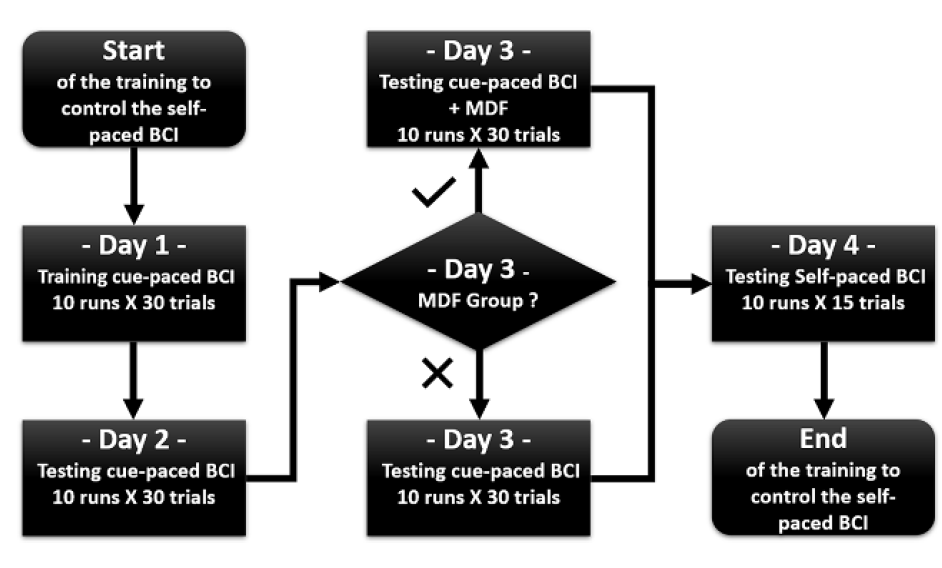
22명의 건강한 사람들이 실험에 참가했으며 총 4일간 진행되었다.
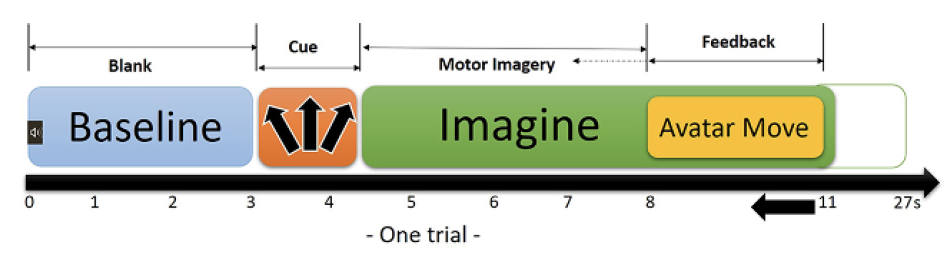
Cue 신호시 VE속 avatat의 152cm 앞에 노란색 arrow가 생성되고 참가자는 이 cue를 보고 MI task를 진행한다.
Day 1. Cue-paced BCI design Training
첫번째 날, 실험은 Classifier를 학습시킬 데이터를 수집하는 것을 목표로 진행된다.
참가자는 cue를보고 MI task를 진행하고 Imagine를 통해 발생하는 EEG신호와 무관하게 VE속 avatar는 움직인다.
수집된 데이터들은 아래 두개의 classifier를 학습시킨다.
- Cl1: two possible classes (walking forward or no movement)
- Cl2: three possible classes (right step, left step or no movement)
Day 2. Cue-paced BCI design Testing
두번째 날, day 1과 같은 paradigm을 갖고 실험이 진행되지만, Imagine를 통한 VE속 avatar의 움직임은 전날 학습된 Classifier의 결과에 따라 움직인다.
avatar는 EEG data에 따라 움직임
이 classifier의 결과는 200ms마다 생성되며, 상태가 빠르게 변하는 것을 방지하기위해 N번 이상 같은 결과일 경우에만 avatar의 상태를 변경한다는 기술을 사용했다.
Day 3. MDF cue-paced BCI design
paradigm은 전과 같으며, 2개의 Classifier들은 day 1,2 data로 재학습된다.
또한 세번째 날부턴 22명의 참가자가 2그룹으로 나뉘고 참가자들에겐 위 사실을 알리지 않는다.
- MDF 그룹: 실험의 70%를 Classifier 결과에 상관 없이 cue에 해당하는 movement로 움직임
- 일반(RGF) 그룹: Classifier 결과에 해당하는 movement로만 움직임
Day 4. Multi-modal self-paced BCI design
기존 실험은 cue-paced로 진행되었지만 Day 4의 경우 매번 cue가 제시되지 않고 avatar가 도달해야하는 목적지와 어떤 mode로 도달해야하는지 제공되는 self-paced로 진행된다.
mode 두가지가 존재하며 아래와 같다.
- continuous mode: avatar가 앞을 향해 움직이도록 상상을 계속 유지하는 mode
- switch mode: 좌/우 step을 상상하여 avatar가 좌우로 움직이면서 앞으로 나아가는 mode
switch mode의 경우 cue는 제시되지만, 해당 cue의 목적은 최적의 경로를 안내하는 네비게이션 역할
C. EEG signal pre-processing
- Data was segmented into 200ms
- $F_{s}$ = 256 Hz
- Band-pass filtered (18th order Butterworth IIR filter) between 8 and 30 Hz
- Artifacts & noise rejection: automatic independent component analysis and noise-rejection algorithm
- signal detrend
D. Feature extraction
- Power spectral density (PSD) of every channel
- 5 PSD asymmetrical ratios (1 s hanning) were chosen as the feature sets
- features were segmented 4~8s using 200 ms epochs with no overlap
- Data were segmented over the 8–30 Hz frequency range using 3 Hz bins with 2 Hz overlap
- The PSDs in each specific segment and frequency range were all concatenated
F. Feature selection
- redundant features를 삭제하기위해 Wilcoxon signed rank based feature selection이 사용됨
- mean absolute value of the cross-correlation coefficient이 계산되었으며 유의하게 다르지 않은 feature는 삭제되었음
G. Classification
Classifier 1,2는 regularized linear discriminate analysis (RLDA)가 사용된다.
Day 4에선 두가지 Classifier가 사용됐다. 먼저 Classifier 1 (Cl1)에 EEG가 입력되어 “no_movement” 결과가 나오면 Classifier 2 (Cl2)에 입력되어 분류되어진다.
H. Performance evaluation
cue paced BCI (days 2, 3) 은 correctly classified trials의 수로 평가되어진다.
self-paced BCI (day 4)의 경우 아래 parameter에 따라 평가되어진다.
- (a) BCI performance accuracy: for each trial, if the participant met all three requirements, it was considered successful.
- (b) Trajectory completion time: the average time it took to complete the trial.
- (c) Average number of stops: during forward walking, the number of times a participant stopped before reaching the target.
- (d) Average stop times: the mean time elapsed between each step, in switch mode.
- (e) Steps alternation performance: the number of times a participant was able to alternate between left and right steps, in switch mode.
- (f) Maximum walk-maintain time: the longest amount of time the participant was able to maintain the MI of forward walking, without any stops or interruptions. All parameters were averaged over the last
I. Spectral map analysis & Questionnaires
최고의 classification accuracy를 갖는 데이터로 Spectral map analysis가 진행되었으며 각 session 이후 설문조사가 진행된다.
Results
A. Retraining of the classifiers—cue-paced BCI
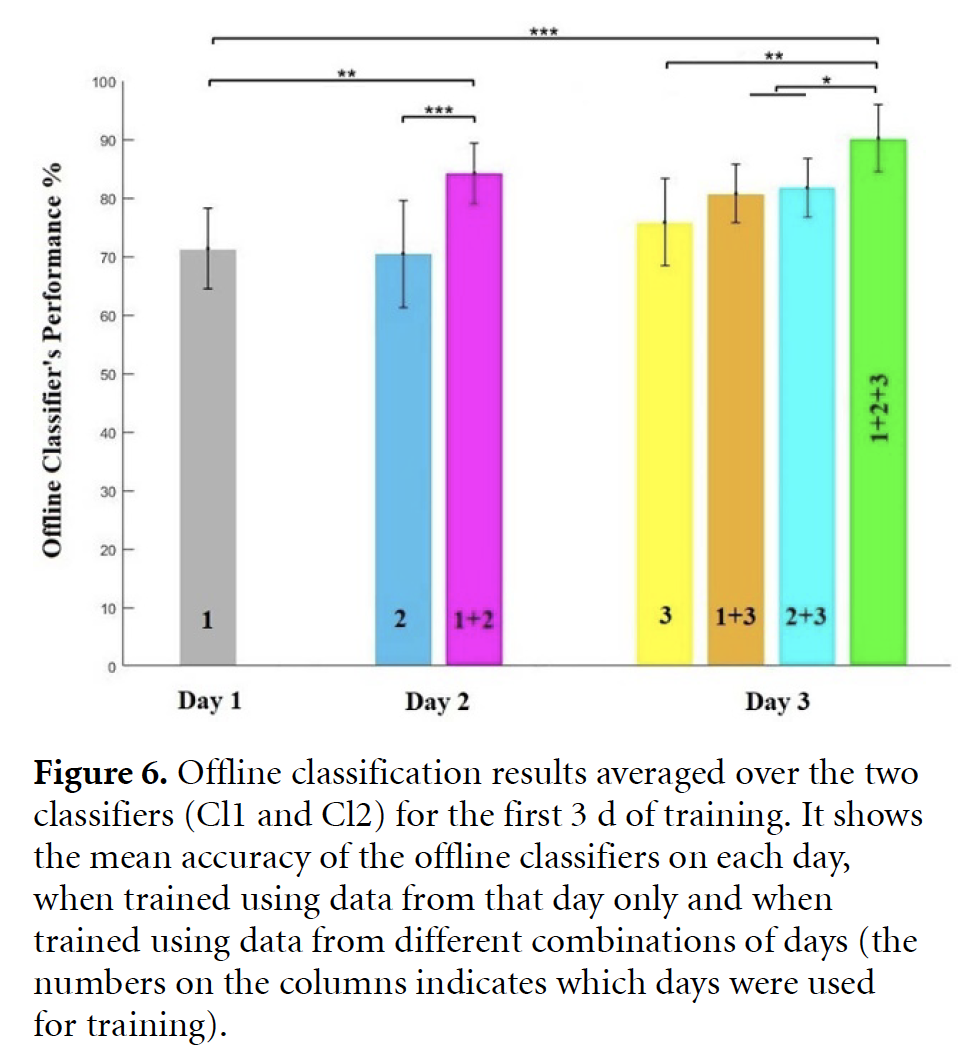
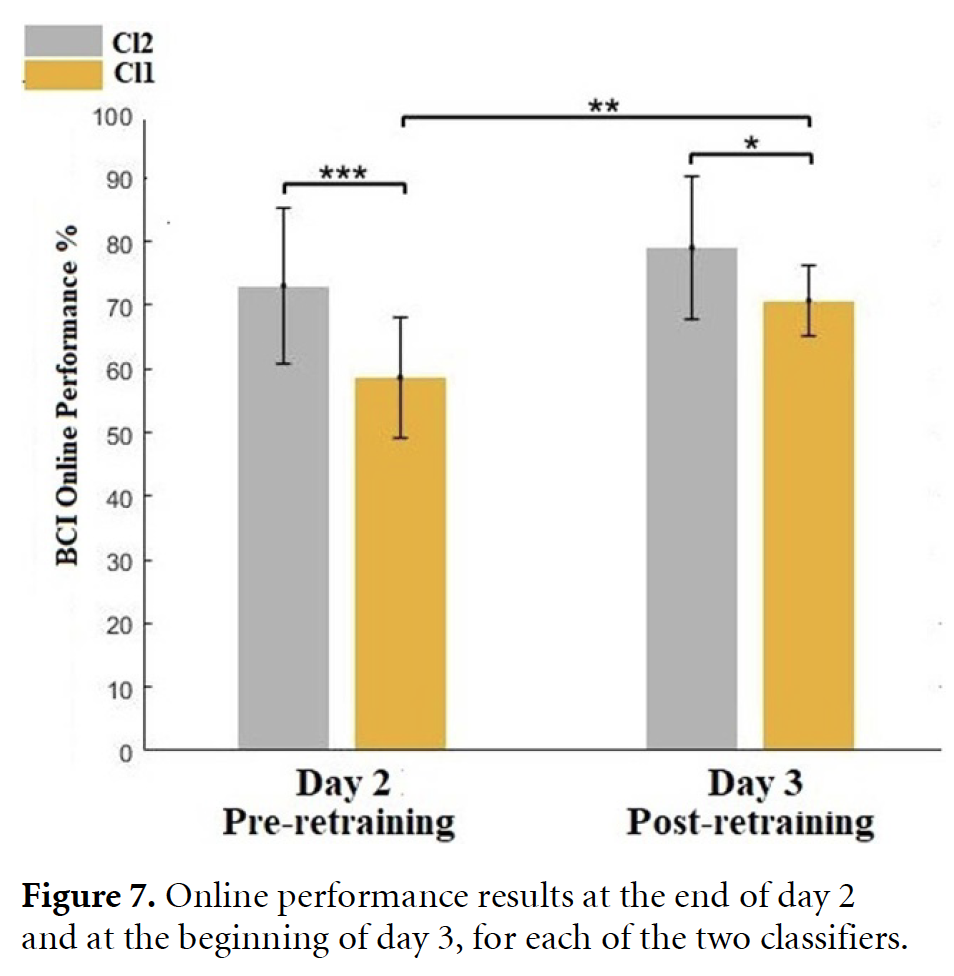
B. MDF—cue-paced BCI
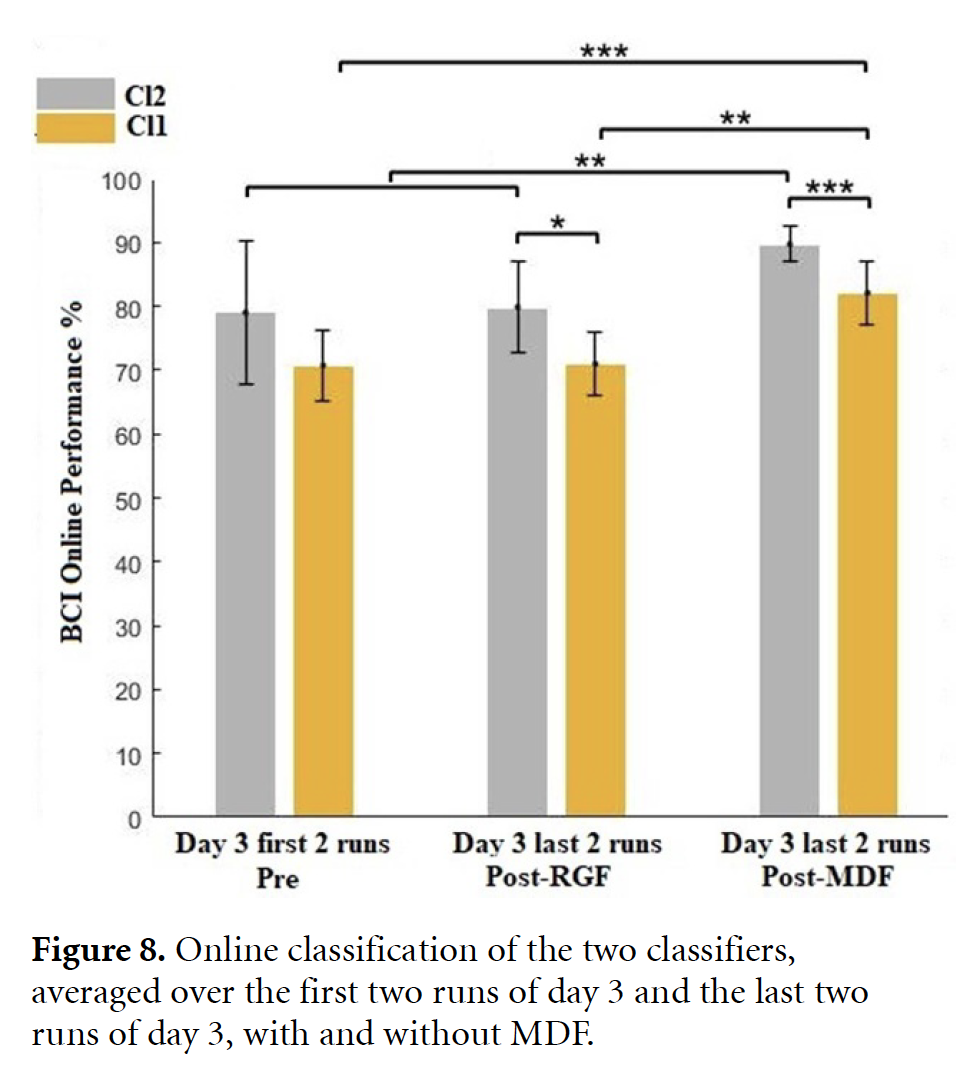
C. MDF—cue-paced BCI: neurophysiological results
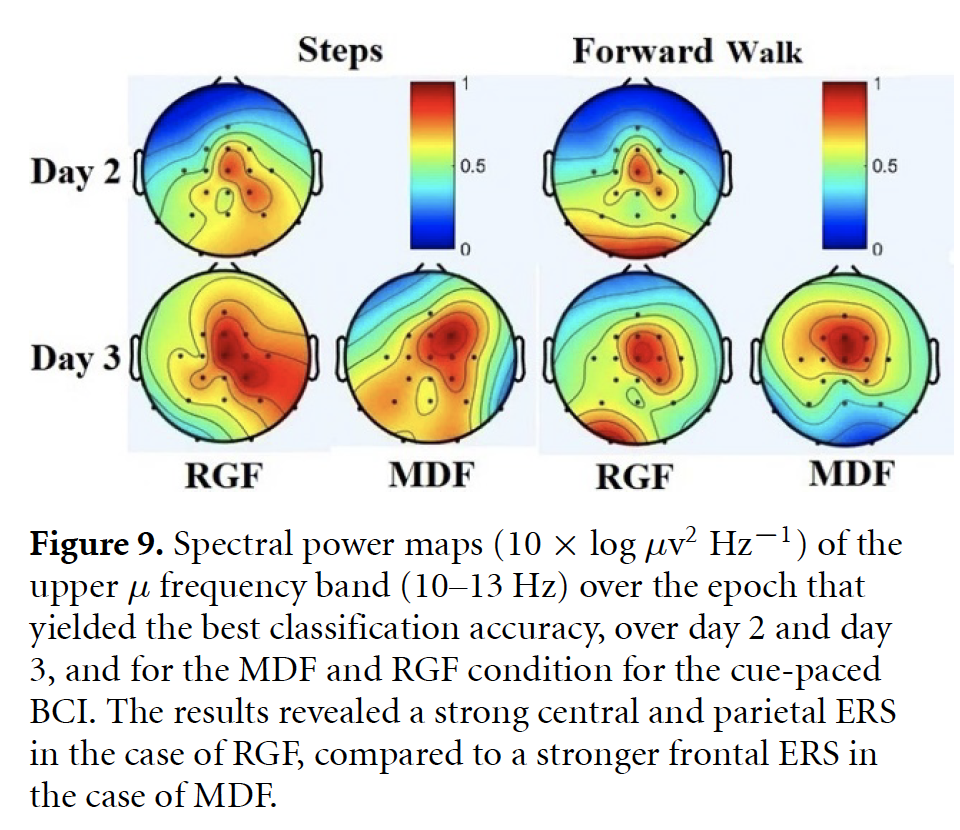
D. Self-paced BCI
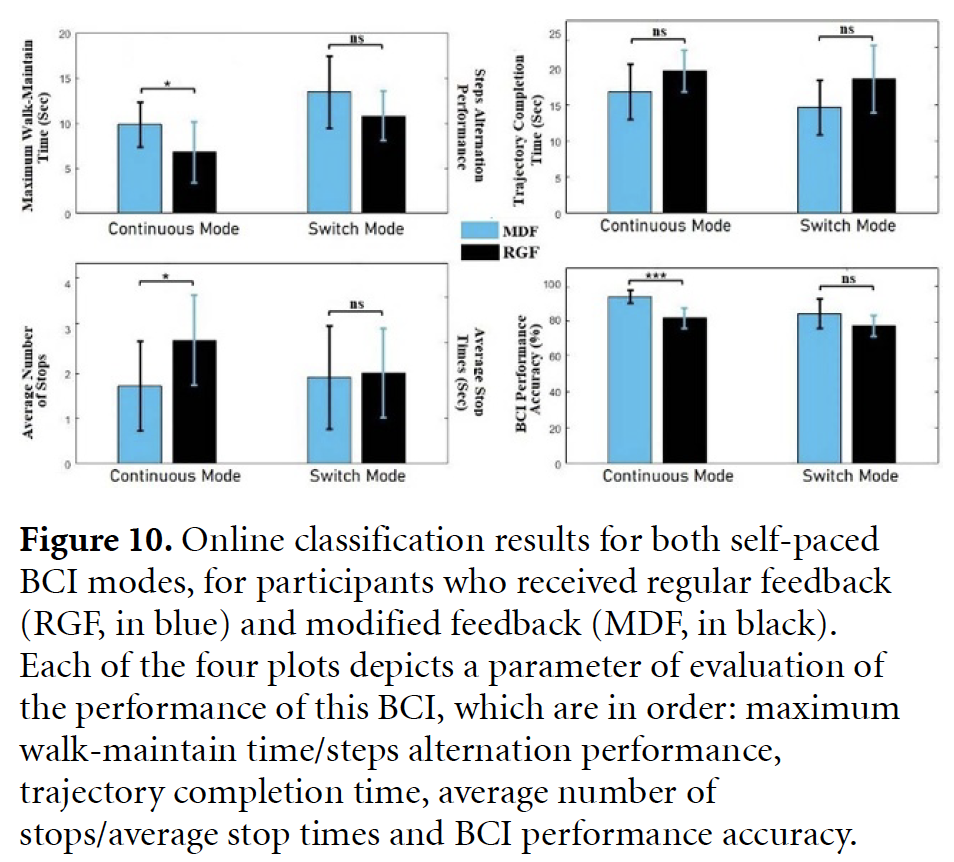
E. Behavioral results
- perceived body ownership: MDF, RGF 둘다 Day 3까지 점차 증가하지만 Day 4에선 RGF만 감소
- perceived sense of agency: MDF가 Day 3부터 증가
- BCI design: MDF, RGF 둘다 증가하자만 MDF가 더 크게 증가
Discussion & Conclusion
이 연구는 VR에서 lower MI를 사용하여 avatar의 움직임을 제어하는 BCI 시스템의 성공적인 설계 및 개발했다.
아래 세가지는 본 BCI-system을 성공적으로 개발할 수 있었던 요소이다.
- Retraining of the classifiers (cue-paced BCI) : 매 seesion마다 학습을 진행함
- MDF (cue-paced BCI): MDF 실험과정을 겪었을 때 더 좋은 결과를 보임
- Co-adaptive sequential training (self-paced BCI): cue-paced BCI로 훈련한 덕에 self-paced BCI에서 더 좋은 결과를 보였음.
본 BCI-system는 주체성을 느낄 수 있는 avatar로부터 시각적 피드백을 얻고, 실제로 걷는 상상과 앞으로 쭉 걷는 상상을 함으로써 보행 재활에 사용될 수 있음을 주장한다.
Reference
- DOI: 10.1088/1741-2552/abee51